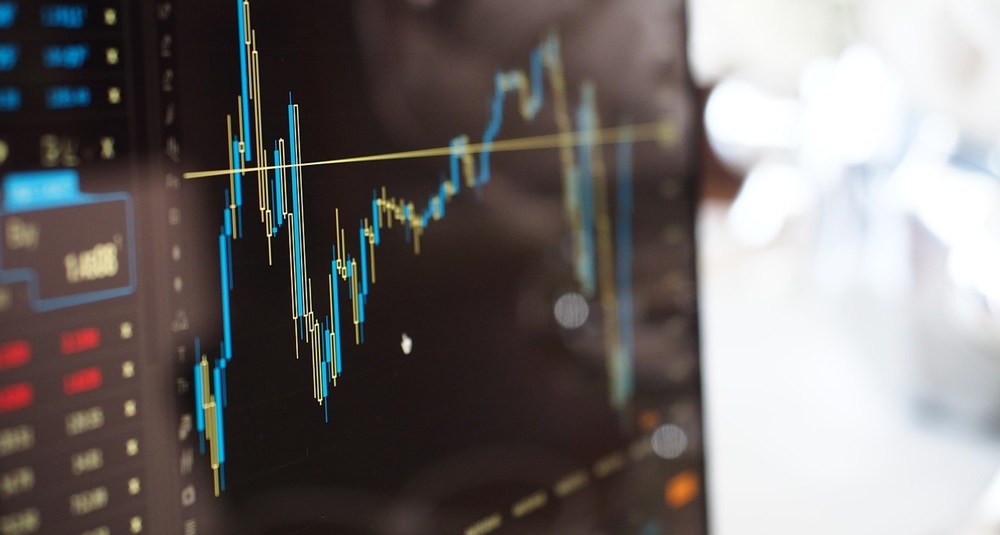
How do you extract the most value from all your Internet of Things (IoT) data?
The IoT steadily generates stunning amounts of data. According to Gartner, 8.4 billion connected things were in use in 2017, up 31 percent from 2016. And they expect this number to reach 20.4 billion by 2020. By 2025, the IoT is projected to generate more than 2 zettabytes of data, says Machina Research.
Manufacturers understand that working with equipment that moves poses different challenges than working with equipment that is stationary. If equipment has multiple moving parts that operate at high speed, the management challenges can be quite complex. Data management is very similar: Data that moves is more challenging to work with than “landed” data sitting quietly in a database. With the high volume and velocity of data constantly streaming in from IoT sensors, you need a different type of data management solution than that required for traditional, stationary transactional data. Two critical differences between management systems for streaming and stationary data include:
- IoT data needs to be managed and analyzed in real time rather than relying exclusively on historical reporting and analysis.
- Streaming IoT data can deliver additional value when analyzed in multiple phases versus once or twice with stationary systems.
Real-time analytics versus after-the-fact
Imagine that you’re driving a truck in Colorado in the middle of winter. Your company has fitted the vehicle with IoT sensors that continually monitor wheel slip, air temperature, speed, and RPMs. Suddenly, the wheel slip measurement spikes as the air temperature falls below zero degrees Celsius. If you can react in milliseconds, you can prevent an accident. If you can’t, the sensor data is meaningless. The data, just like the vehicle, is in motion.
Event stream processing systems can enable you to act on this information in a timely fashion through real-time data cleansing and analytics.
When event stream processing systems manage data from IoT sensors, they perform processes that turn raw data into useful information in real time. As large amounts of data rapidly stream into the system, event stream processing systems cleanse, normalize and aggregate data immediately in-memory. Simultaneously, real-time analytics models encoded in these data streams perform analysis to determine whether a specific event is relevant and generate instant alerts when urgent action is needed.
When the truck is in danger of slipping on the icy road, real-time analytics at the edge immediately alert the driver to slow it down.
Event stream processing systems also filter data in real time. Because the memory in which these systems initially store data is limited, the event stream processing system decides what data to discard and what to keep long term.
If a sensor is tracking temperature and the temperature stays steady, the system doesn’t store ongoing readings. Instead, it might retain only the readings that indicate a change.
In contrast, traditional relational database management (RDBMS) systems store all data and perform cleansing and analysis after the fact. RDBMS systems collect data from predefined sources and store it in a persistent storage system, such as a data mart. Once in storage, data is cleansed, normalized and consolidated into a data warehouse or Hadoop. Only then can users derive meaning from the data through reporting, historical analysis—and even predictive analysis and machine learning.
Multi-phase analytics
Another difference between event stream processing and traditional, stationary data analytics is that the former gives you multiple opportunities to extract value from your data. With traditional data management, data is historical and does not change. It may be analyzed once or twice after the fact, not more.
As discussed previously, event stream processing systems first analyze data in real time enabling immediate response to events. Just as motion applications in manufacturing look at different axes of movement, modern analytics systems look at different “axes” of relevance and maximize the limited storage capacity and bandwidth for what is most important. In real-time or near real time, you can bring a subset of the data from multiple sensors back to the cloud or on-premises for cross-sensor analysis.
You might perform analysis across your entire fleet of trucks to determine fault conditions occurring at a certain elevation. If the system detects a problem, it could trigger a mass work order for repair of all affected trucks in the fleet.
Finally, the event stream processing system also stores specified data in a data warehouse or Hadoop. There you can perform visual analytics or visual statistics on the historical data now that the data is stationary and easier to manage.
In this case, you could use machine learning algorithms for predictive maintenance. Over time, machine learning algorithms can learn patterns that indicate when trucks will soon require maintenance to catch failures before they happen.
In all steps of the multi-phase analytics, machine learning can train the system to better predict outcomes. As the model changes, the stream processing solution can update the models at the edge, on-premises or in the cloud when necessary.
Management systems for streaming data allow you to glean meaning from IoT data when you need it. Not only can you use event stream processing systems to respond to events in real time, you can also perform multiple rounds of cross-sensor and historical analysis to wring the ultimate information goodness from all your sensor data.
About the author: Marcia Elaine Walker is the Principal Industry Consultant for Manufacturing at SAS. Follow her on LinkedIn or @MWEnergy on Twitter. Follow SAS news @SASsoftware on Twitter.
Edited by
Ken Briodagh